Keith Giglia
- MRIWA PhD Scholarship Alumnus
- 2023 MRIWA PhD Graduate
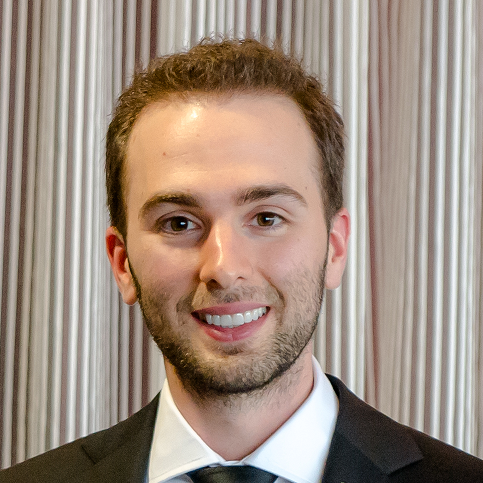
Project
Monitoring and control of hydrocyclones by use of convolutional neural networks and deep reinforcement learning
Host University
Curtin University
The Challenge
Hydrocyclones are complex apparatus controlling material flow in many modern mineral processing systems. Their efficient operation can deliver significant efficiency and cost benefits, but hydrocyclones are typically monitored only at a cluster level, and operated with low-level control.
Key Findings
- Image-based hydrocyclone monitoring using convolutional neural networks and model-free learning delivered significant performance benefits.
- Trained systems were able to distinguish between correct and fault states under industrial conditions
- This performance provides the basis for automated monitoring and control of hydrocyclone circuits, identifying obstruction or suboptimal performance and modifying operating conditions accordingly.
Benefits to WA
Keith’s research paves the way to automation of hydrocyclone operation in mineral processing and related material industries. Remote monitoring and control of this critical infrastructure could deliver important performance improvements in terms of both efficiency and safety to the WA mineral processing industry.
Link to thesis
Keith’s thesis can be accessed in the Curtin collection via the link below:
Page was last reviewed 22 September 2023